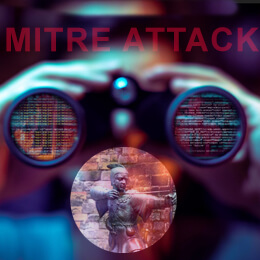
Preventing Zero Day Attacks using MITRE ATT&CK Framework

By Kobi Eisenkraft and Asaf Fried, Threat Prevention R&D
This is the third installment of a three-part series about how Check Point employs the MITRE ATT&CK framework to prevent cyberattacks. Read Part 1 and Part 2.
The challenge
Check Point sandboxing technology determines what MITRE ATT&CK technique the malware uses during threat emulation. This helps investigate the malware’s behavior.
Our challenge is then to take this one step further to prevent attacks before they happen using the techniques observed during threat emulation.
The technology
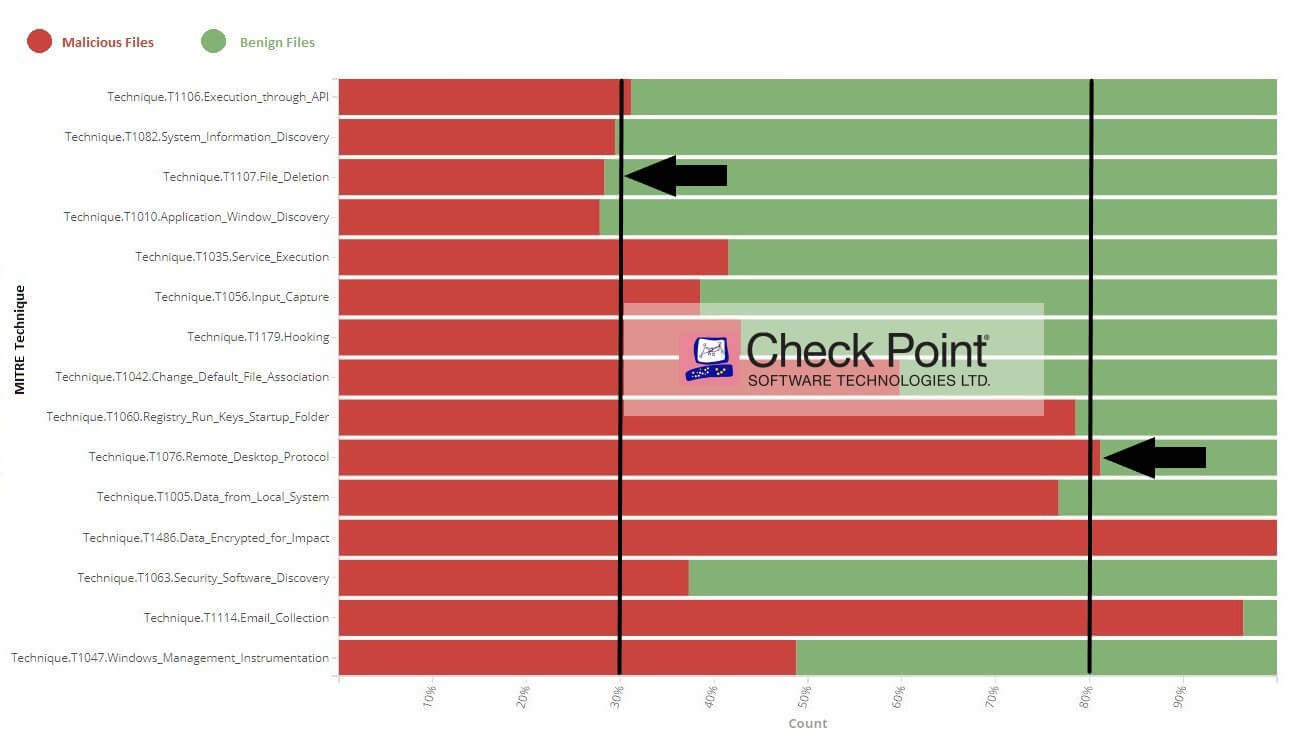
Figure 1: Top 15 techniques used by malware, highlighting the maliciousness of each.
Figure 1 shows the top 15 techniques a malware uses and indicates the level of maliciousness for each technique. We can see from this chart that all the techniques are observed, however each has different levels of frequency and maliciousness. For example, more than 80% of the files that used Remote Desktop Protocol (T1076) are malicious. In contrast, fewer than 30% of the files that used File Deletion (T1107) are malicious.
Using Check Point’s telemetry data, we assigned a score for each technique based on the probability of the techniques used in benign and malicious files. However, we didn’t stop there. Instead, we went one step further and issued a score for each file based on all the techniques that were used.
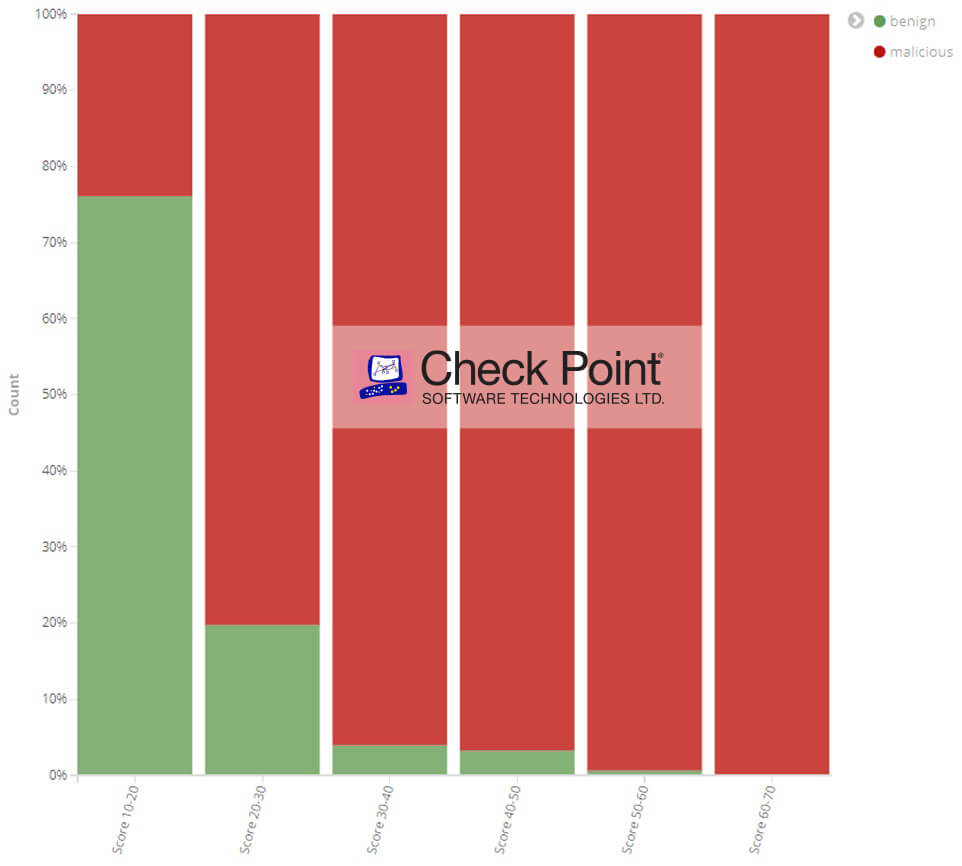
Figure 2: Check Point’s scoring system for files using MITRE ATT&CK
Now that we have scores for both the techniques and the files, we wanted to answer the question:
“Can this data be used for prevention of malicious files using data mining and machine learning tools?”
In order to do that, we created and trained a machine learning model based on the MITRE ATT&CK data from over 100,000 unique files.
Preventing attacks using the MITRE framework
We monitored the findings after deploying the model. The model accurately detected known malicious files. However, we were interested in the malicious files that were unknown and not well detected by other available solutions, such as files with a low catch rate on VirusTotal.
At the time we wrote this blog the model detected a malicous sample with a relatively low detection rate 12/69 (~17%) by VirusTotal.
Sha256: 65c424deb94c43c003a66c88736edd21875bae07282e1d78d32116d12da4ebe4

Figure 3: VirusTotal scan results for a sample with a low detection rate.
The malicious sample utilized seven tactics with 15 different techniques, which is significant for a file with a low detection rate by other solutions. The combination of all of these techniques led our model to categorize the file malicious with high confidence, and prevent it from reaching our customers.
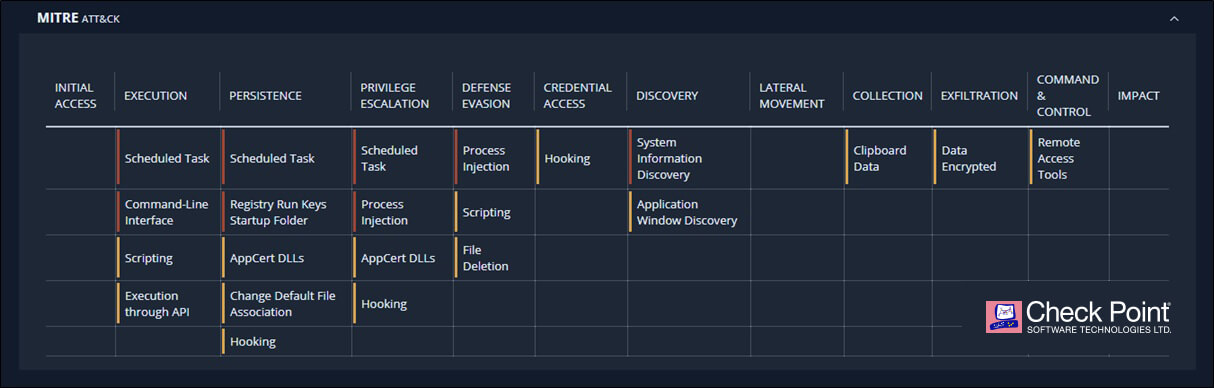
Figure 4: The sample MITRE ATT&CK Matrix using SandBlast Network.
Upon closer analysis of the malware, we realized we’re looking at the Remcos phishing campaign. Our machine learning model prevented this unknown threat by using only the MITRE ATT&CK data.
Conclusion
In Part 1 and Part 2 of this three part series we showed that the MITRE ATT&CK framework is important as a common shared language among cybersecurity practitioners. We have also demonstrated that by leveraging Check Point’s logs with MITRE ATT&CK data, we are providing analysts and incident response teams a new platform to analyze threats in order to respond and quarantine threats quickly.
In this final part, we discuss that by combining the MITRE ATT&CK framework with machine learning engines, we have created a very powerful tool for both cyber threat intelligence and prevention.
So keep calm and let Check Point’s SandBlast Network secure your data with our new MITRE ATT&CK machine learning engine.