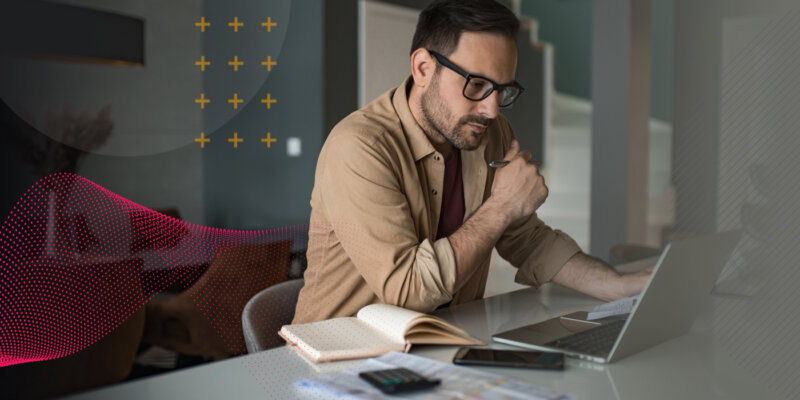
DeepBrand Clustering – an Evolution in Brand Spoofing Prevention

Phishing remains a significant component of the cyber threat landscape due to its simplicity, effectiveness, and adaptability. It is a deceptive practice in which threat actors pose as legitimate entities in an effort to extract sensitive information from unsuspecting individuals.
The prevalence of phishing is attributed to its low-cost execution and high success rate, especially as digital communication becomes more integral to daily life.
Phishing tactics have evolved, with variations like spear-phishing, whaling, smishing, and more. It continues to be a top tool for cybercriminals because it exploits the most vulnerable element of security systems: human psychology. Phishing is so prolific that a whopping 94% of organizations reported falling victim to it in 2023.
Last year, we introduced a groundbreaking technology called “Brand Spoofing Prevention,” a preemptive engine within ThreatCloud AI designed to prevent both global and local brand impersonation attacks. This technology uses advanced technologies, such as AI, Natural Language Processing (NLP), image processing, and heuristics, to detect and prevent attempts of brand impersonation by matching URLs and web pages with established brands.
Our new DeepBrand Clustering technology is the next evolution of Brand Spoofing Prevention, designed to keep up with the growing number of websites and spoofed pages.
The Digital Brand Challenge
Identifying and indexing every brand on the internet is an unsustainable task aimed at finding a needle in a constantly expanding haystack. The volume of brand websites makes detecting brand spoofing challenging, leaving many attempts undetected and exposing consumers and businesses to fraud and cyberattacks. Hence, there’s a pressing need for automated, intelligent systems that can adapt and scale with the growing digital brand ecosystem.
A major challenge in detecting brand spoofing scams is labeling data needed to train the relevant AI models. This requires identifying diverse brand elements and understanding nuanced differences between them. It’s a labor-intensive and complex process, complicated by the dynamic nature of branding.
Achieving precision at scale is difficult. Both labeling and developing heuristics are not feasible, making supervised ML models irrelevant.
To tackle data labeling, we turned to unsupervised learning, automatically attributing web page characteristics to brands. This approach reduces reliance on human intervention, saving time and minimizing errors in brand element identification.
DeepBrand Clustering – Patent-Pending AI Engine Built for Scale
The solution unfolds in two phases: learning and incrimination.
Learning
DeepBrand Clustering constructs a neural network using attributes extracted from observed web pages sourced from Check Point’s global traffic.
DeepBrand Clustering represents an innovative unsupervised learning model that combines the power of Deep Neural Networks (DNNs) with traditional machine learning (ML) models. By integrating advanced approaches from the fields of artificial intelligence and cybersecurity, DeepBrand Clustering achieves cutting-edge results.
The neural network trains on unlabeled traffic in order to learn to identify brands automatically and without supervision, based on common characteristics in the web page, such as domain, favicon, title, and more.
In order to train this model, we have defined a pipeline that consists of multiple steps. These steps range from extracting brand indicators to automatically assigning brand names to clusters. Some steps focus on collecting visual or text indicators, while others handle data transformation. Additionally, certain components of this pipeline involve deep neural networks (DNNs) trained using advanced augmentation techniques based on domain knowledge from cybersecurity approaches.
Once data is gathered and standardized The output of the entire pipeline is a trained model (ready for inference) with multiple distinct clusters and assigned brand names, the model organizes web pages into clusters associated with specific brands, and each cluster is labeled accordingly. These clusters, particularly the most distinct ones, are utilized to analyze real-time traffic and identify brand presence.
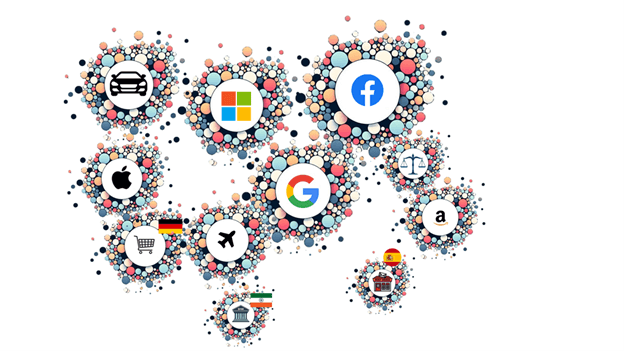
Figure 1 – Illustration of how DeepBrand Clustering constructs a neural network using attributes extracted from observed web pages
Incrimination
This innovation enables an expanded incrimination engine. During the incrimination phase, an inference process determines whether the examined web page belongs to any of the established clusters. If so, the engine evaluates whether the activity signifies a potential malicious brand spoofing attempt
This technique represents a significant leap forward in brand protection technology. The entire system is patent pending, underscoring its novel approach and the advanced capabilities it brings to the challenge of brand spoofing detection.
Unparalleled Brand Spoofing Protection
Within several hours of running the learning phase, DeepBrand clustering indexed more than 4000 distinct brands. In the past 30 days, 75% of the indexed brands (3700) were observed in Check Point traffic. Out of the observed brands, more than 200 unique brands were spoofed in more than 4000 malicious attacks. Specifically, we detected 975 instances across 101 local brands.
The new DeepBrand Clustering engine protected more than 210 customers from more than 190 countries worldwide.
The landscape of brand spoofing attacks is constantly evolving, with new threats emerging frequently. DeepBrand Clustering’s enhanced detection capabilities allow it to be at the forefront, often identifying brand spoofing attacks before they are even known and added to databases like VirusTotal.
Check Point’s Zero-Phishing engine, part of ThreatCloud AI, revolutionizes Threat Prevention, providing industry leading security as part of Check Point’s Quantum, Harmony and CloudGuard product lines.
To learn about Check Point threat prevention, schedule a demo or a free security checkup to assess your security posture.